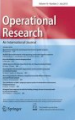
Stock control analytics: a data-driven approach to compute the fill rate considering undershoots
Eugenia Babiloni and Ester Guijarro and Juan R. Trapero
Operational Research, 2023
One of the most frequently used inventory policies is the order-point, order-up-to level (s, S) system. In this system, the inventory is continuously reviewed and a replenishment request is placed whenever the inventory position drops to or below the order point, s. The variable replenishment order quantity and the variable replenishment cycle characterize the system by the use of complex mathematical computations. Diferent methodological approaches diminish the mathematical complexity by neglecting the undershoots, i.e., the quantity that the inventory position is below the order point when it is reached. In this paper, we conceptually and empirically
analyse the bias that neglecting the undershoots introduces into the estimation of the fll rate. After that, we suggest a new methodology developed under a data-driven perspective that uses a state-dependent parameter algorithm to correct such a bias. As a result, we propose two new methods, one parametric and the other nonparametric, to enhance the fll rate estimate. Both methods, named analytics fll rate methods, remove the bias that neglecting the undershoots introduces and are used to illustrate the practical implications of this hypothesis on the performance and design of the (s, S) system. This research is developed in a lost sales context with simulated stochastic and i.i.d. discrete demands as well as actual sales data.
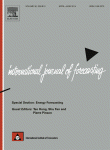
Forecasting: theory and practice
Fotios Petropoulos and Daniele Apiletti and Vassilios Assimakopoulos and Mohamed Zied Babai and Devon K. Barrow and Souhaib Ben Taieb and Christoph Bergmeir and Ricardo J. Bessa and Jakub Bijak and John E. Boylan and Jethro Browell and Claudio Carnevale and Jennifer L. Castle and Pasquale Cirillo and Michael P. Clements and Clara Cordeiro and Fernando Luiz Cyrino Oliveira and Shari De Baets and Alexander Dokumentov and Joanne Ellison and Piotr Fiszeder and Philip Hans Franses and David T. Frazier and Michael Gilliland and M. Sinan Gönül and Paul Goodwin and Luigi Grossi and Yael Grushka-Cockayne and Mariangela Guidolin and Massimo Guidolin and Ulrich Gunter and Xiaojia Guo and Renato Guseo and Nigel Harvey and David F. Hendry and Ross Hollyman and Tim Januschowski and Jooyoung Jeon and Victor Richmond R. Jose and Yanfei Kang and Anne B. Koehler and Stephan Kolassa and Nikolaos Kourentzes and Sonia Leva and Feng Li and Konstantia Litsiou and Spyros Makridakis and Gael M. Martin and Andrew B. Martinez and Sheik Meeran and Theodore Modis and Konstantinos Nikolopoulos and Dilek Önkal and Alessia Paccagnini and Anastasios Panagiotelis and Ioannis Panapakidis and Jose M. Pavía and Manuela Pedio and Diego J. Pedregal and Pierre Pinson and Patrícia Ramos and David E. Rapach and J. James Reade and Bahman Rostami-Tabar and Michał Rubaszek and Georgios Sermpinis and Han Lin Shang and Evangelos Spiliotis and Aris A. Syntetos and Priyanga Dilini Talagala and Thiyanga S. Talagala and Len Tashman and Dimitrios Thomakos and Thordis Thorarinsdottir and Ezio Todini and Juan Ramón Trapero Arenas and Xiaoqian Wang and Robert L. Winkler and Alisa Yusupova and Florian Ziel
International Journal of Forecasting, 2022
Publication year: 2022
Forecasting has always been at the forefront of decision making and planning. The uncertainty that surrounds the future is both exciting and challenging, with individuals and organisations seeking to minimise risks and maximise utilities. The large number of forecasting applications calls for a diverse set of forecasting methods to tackle real-life challenges. This article provides a non-systematic review of the theory and the practice of forecasting. We provide an overview of a wide range of theoretical, state-of-the-art models, methods, principles, and approaches to prepare, produce, organise, and evaluate forecasts. We then demonstrate how such theoretical concepts are applied in a variety of real-life contexts.
We do not claim that this review is an exhaustive list of methods and applications. However, we wish that our encyclopedic presentation will offer a point of reference for the rich work that has been undertaken over the last decades, with some key insights for the future of forecasting theory and practice. Given its encyclopedic nature, the intended mode of reading is non-linear. We offer cross-references to allow the readers to navigate through the various topics. We complement the theoretical concepts and applications covered by large lists of free or open-source software implementations and publicly-available databases.
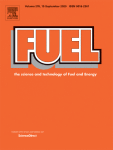
Impact of the forecast price on economic results for methanol production from olive waste
M. Puig-Gamero and J.R. Trapero and D.J. Pedregal and P. ánchez and L. Sanchez-Silva
Fuel, 295: 120631
Publication year: 2021
The development of circular economies due to the limitation of natural resources is becoming a common strategy of paramount importance among different countries. In Spain, given the strategic nature of its olive industry, trying to value one of its main residuals (olive pomace) alone or together with other residues through its chemical transformation in methanol is a promising research line. One of the key variables that would make the investment advisable or not is the future value of the methanol price. However, most of the literature do not consider its future price volatility on the economic evaluation of the chemical processes. This work bridges that gap by proposing three econometric models based on Unobserved Components to forecast the methanol price over the life cycle plant. Those probabilistic forecasts feed a Monte Carlo simulation that provides an exhaustive investment risk assessment in terms of Net Present Value, Internal Rate of Return and Value at Risk metrics. The results showed the relationship between forecasting models and the investment profitability with an average Internal Rate of Return ranging from 23% to 31%. Additionally, the previous analysis was completed by adding other variables subject to uncertainty (olive pomace feed, capital investment, feedstock price, labor costs and discount rate). In this case, assuming a potential underestimation error up to 100% of the capital cost, the probability of obtaining a profitable investment was significantly reduced ranging the Value at Risk from 48% to 98%.
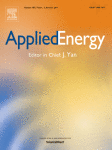
Adjusted combination of moving averages: A forecasting system for medium-term solar irradiance
Diego J. Pedregal and Juan R. Trapero
Applied Energy, 298: 117155
Publication year: 2021
Global Horizontal Irradiation forecasts are necessary for an efficient use of fluctuating energy output from photovoltaic plants. The purpose of this paper is to provide efficient, easy-to-implement Global Horizontal Irradiation forecasts on an hourly basis for a medium-term horizon (longer than 48 h). These forecasts are essential for the strategic deployment of tasks including unit commitment, transmission management, trading, hedging, planning, asset optimization, maintenance scheduling and spinning of power units. Nonetheless, forecasting models for medium term horizons are scarce. This work intends to bridge that gap by proposing a method based on an adjusted combination of moving averages which includes the yearly cycle in addition to the day–night cycle. This method is straightforward to implement and easy to integrate into corporate computing systems. We compare our approach with several well-known alternative methods, such as deep recurrent neural networks or autoregressive integrated moving average models, among others. The results show that recurrent neural networks are 10% more accurate than the second best method, and 90% better than our proposal for very short horizons (up to 5 h ahead). However, this advantage is lost when longer horizons are tested, especially for horizons longer than 30 h ahead, for which our method produces forecasts which are 20% more accurate than those made by recurrent neural networks. The reason behind that improvement at longer horizons is the inclusion of the yearly cycle in the proposed approach.
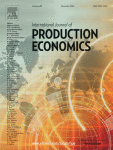
Optimising forecasting models for inventory planning
Nikolaos Kourentzes and Juan R. Trapero and Devon K. Barrow
International Journal of Production Economics, 225: 107597,
Publication year: 2020
Inaccurate forecasts can be costly for company operations, in terms of stock-outs and lost sales, or over-stocking, while not meeting service level targets. The forecasting literature, often disjoint from the needs of the forecast users, has focused on providing optimal models in terms of likelihood and various accuracy metrics. However, there is evidence that this does not always lead to better inventory performance, as often the translation between forecast errors and inventory results is not linear. In this study, we consider an approach to parametrising forecasting models by directly considering appropriate inventory metrics and the current inventory policy. We propose a way to combine the competing multiple inventory objectives, i.e. meeting demand, while eliminating excessive stock, and use the resulting cost function to identify inventory optimal parameters for forecasting models. We evaluate the proposed parametrisation against established alternatives and demonstrate its performance on real data. Furthermore, we explore the connection between forecast accuracy and inventory performance and discuss the extent to which the former is an appropriate proxy of the latter.
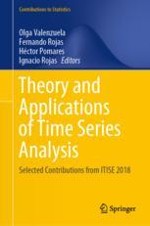
On the Automatic Identification of Unobserved Components Models
Pedregal, Diego J. and Trapero, Juan R.
In Theory and Applications of Time Series Analysis
Publication year: 2020
Automatic identification of time series models is a necessity once the big data era has come and is staying among us. This has become obvious for many companies and public entities that have passed from a crafted analysis of each individual problem to handle a tsunami of information that has to be processed efficiently, online and in record time. Automatic identification tools have never been tried out on Unobserved Components models (UC). This chapter shows how information criteria, such as Akaike’s or Schwarz’s, are rather useful for model selection within the UC family. The difficulty lies, however, on choosing an appropriate and as general as possible set of models to search in. A set too narrow would render poor forecast accuracy, while a set too wide would be highly time consuming. The forecasting results suggest that UC models are powerful potential forecasting competitors to other well-known methods. Though there are several pieces of software available for UC modeling, this is the first implementation of an automatic algorithm for this class of models, to the best of the author’s knowledge.
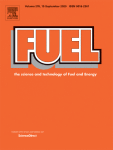
Is methanol synthesis from co-gasification of olive pomace and petcoke economically feasible?
M. Puig-Gamero and J.R. Trapero and P. Sánchez and L. Sanchez- Silva
Fuel, 278: 118284
Publication year: 2020
An economic assessment of methanol production from syngas obtained by co-gasification of petcoke and olive pomace was performed. This process was simulated with Aspen Plus® software. Net Price Value (NPV), Internal Rate of Return (IRR), Payback period (PBP), break-even and minimum product sales price were the techno-economic parameters used for analyzing the economic viability of the project. Firstly, the influence of the blend in co-gasification was analysed to study possible synergies from an economic point of view. The financial results obtained confirmed that the higher the amount of olive pomace, the more financially feasible it was. In addition, a negative NPV was obtained when the petcoke content in the blends was higher than 50 wt%. Moreover, the influence of plant size on economic viability was also analysed. Additionally, a sensitivity analysis was performed with respect to investment, sales price of methanol and methanol production, and the economic results were seen to be significantly affected by the price of methanol. To incorporate the potential risks of methanol price volatility, several Monte Carlo simulations were defined based on density forecasting for the methanol price for the time horizon of the life cycle of the plant. The main results indicated that it was highly probable the plant would be profitable despite the volatility in methanol prices.
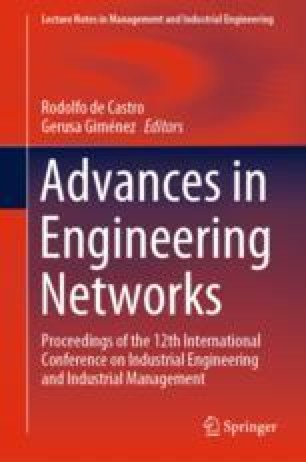
A Non-parametric Enhancement of the Fill Rate Estimation
Eugenia Babiloni, Ester Guijarro, Juan R. Trapero
In Advances in Engineering Networks, 2020
Publication year: 2020
One of the main objectives of an inventory system consists of guarantee a target service level. However, classical approaches are based on assumptions that introduce significant errors. This fact can lead to design inventory policies that do not guarantee the achievement of the target service level. This paper suggests an expression to compute the fill rate for the continuous base-stock policy based on the classic derivation that neglects undershoots at the reorder point. Subsequently, the error incurred for neglecting the undershoots is calculated and corrected by means of the state-dependent parameter approach expressed in a state space framework. The results show that the empirical approach reduces the classic fill rate bias. Simulation is employed to corroborate these results.
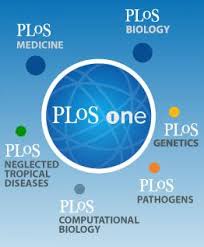
A literature review on operational decisions applied to collaborative supply chains
Holgado de Frutos, Enrique AND Trapero, Juan R. AND Ramos, Francisco
PLOS ONE, 15(3): 1—28
Publication year: 2020
Throughout the last decades, collaborative schemes, under an amalgam of different acronyms (ECR, CPFR, VMR, etc.), have been developed to mitigate the problematic Bullwhip effect. Essentially, companies work together by either sharing information, making joint decisions, or sharing benefits to reach potential synergies. This work aims at reviewing these works through a systematic literature review process to investigate the different collaborative models from an operational perspective. A total of 92 articles have been classified into 3 categories: Information Exchange; Vendor Managed Replenishment; and Synchronized Supply Chain. For each category, we have identified the type of research, supply chain structures, forecasting models, demand characteristics, replenishment policies and assumptions employed in the considered articles. This article identifies the main results achieved and the gaps and opportunities to be developed as further research.
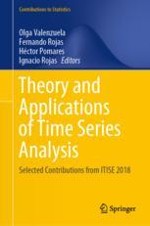
Time Series Modeling with MATLAB: The SSpace Toolbox
Pedregal, Diego J. and Villegas, Marco A. and Villegas, Diego A. and Trapero, Juan R.
In Theory and Applications of Time Series Analysis, 2019
Publication year: 2019
SSpace is a MATLAB toolbox for State-Space modeling that provides the user with tools for linear Gaussian, nonlinear, and non-Gaussian systems with the most advanced and up-to-date features available in any State-Space framework. Great flexibility is achieved because each model is coded on a standard MATLAB function, thence having absolute control on particular parameterizations, parameter constraints, time variation of parameters or variances, arbitrary nonlinear relations with inputs, time aggregation, nested models, system concatenation, etc. The toolbox may be used by specifying State-Space systems from scratch or by using ready-to-use templates for standard methods (like VARMAX, exponential smoothing, unobserved components, Dynamic Linear Regression, etc.). The toolbox is freely available via a public code repository with full documentation and help system. This chapter demonstrates the toolbox’s potential with several examples.
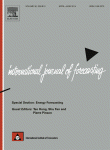
Quantile forecast optimal combination to enhance safety stock estimation
Juan R. Trapero and Manuel Cardós and Nikolaos Kourentzes
International Journal of Forecasting, 35(1): 239—250
Publication year: 2019
The safety stock calculation requires a measure of the forecast error uncertainty. Such errors are usually assumed to be Gaussian iid (independently and identically distributed). However, deviations from iid lead to a deterioration in the performance of the supply chain. Recent research has shown that, contrary to theoretical approaches, empirical techniques that do not rely on the aforementioned assumptions can enhance the calculation of safety stocks. In particular, GARCH models cope with time-varying heterocedastic forecast error, and kernel density estimation does not need to rely on a determined distribution. However, if the forecast errors are time-varying heterocedastic and do not follow a determined distribution, the previous approaches are inadequate. We overcome this by proposing an optimal combination of the empirical methods that minimizes the asymmetric piecewise linear loss function, also known as the tick loss. The results show that combining quantile forecasts yields safety stocks with a lower cost. The methodology is illustrated with simulations and real data experiments for different lead times.
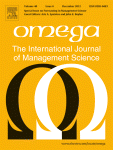
Empirical safety stock estimation based on kernel and GARCH models
Juan R. Trapero and Manuel Cardós and Nikolaos Kourentzes
Omega, 84: 199—211
Publication year: 2019
Supply chain risk management has drawn the attention of practitioners and academics alike. One source of risk is demand uncertainty. Demand forecasting and safety stock levels are employed to address this risk. Most previous work has focused on point demand forecasting, given that the forecast errors satisfy the typical normal i.i.d. assumption. However, the real demand for products is difficult to forecast accurately, which means that—at minimum—the i.i.d. assumption should be questioned. This work analyzes the effects of possible deviations from the i.i.d. assumption and proposes empirical methods based on kernel density estimation (non-parametric) and GARCH(1,1) models (parametric), among others, for computing the safety stock levels. The results suggest that for shorter lead times, the normality deviation is more important, and kernel density estimation is most suitable. By contrast, for longer lead times, GARCH models are more appropriate because the autocorrelation of the variance of the forecast errors is the most important deviation. In fact, even when no autocorrelation is present in the original demand, such autocorrelation can be present as a consequence of the overlapping process used to compute the lead time forecasts and the uncertainties arising in the estimation of the parameters of the forecasting model. Improvements are shown in terms of cycle service level, inventory investment and backorder volume. Simulations and
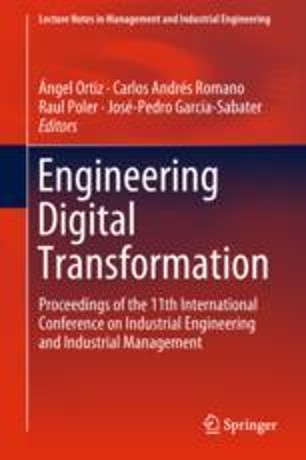
A Simulink Library for Supply Chain Simulation
Trapero, Juan Ramón and Ramos, Francisco and Holgado de Frutos, Enrique
In Engineering Digital Transformation, 343-350
Publication year: 2019
Despite the links between forecasting and inventory control, both areas have evolved separately. This work presents a supply chain simulation library developed in Simulink to bridge that gap. To simulate a supply chain is important to define the number of companies/echelons that belong to the same supply chain and the policies that each company employs to demand forecasting and stock control. The potential user can find in this library forecasting and stock control blocks to simulate a supply chain in a modular design. We show how to implement: i) Forecasting policies. For example, the widely used exponential smoothing; ii) Replenishment models. For instance, typical order-up-to-level stock control policies as (s, S); and finally, how to connect the forecasting and stock control blocks to describe the performance of a company and to extend such relationships to define the whole supply chain.
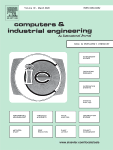
A support vector machine for model selection in demand forecasting applications
Marco A. Villegas and Diego J. Pedregal and Juan R. Trapero
Computers & Industrial Engineering, 121: 1—7
Publication year: 2018
Time series forecasting has been an active research area for decades, receiving considerable attention from very different domains, such as econometrics, statistics, engineering, mathematics, medicine and social sciences. Moreover, with the emergence of the big data era, the automatic identification with the appropriate techniques remains an intermediate compulsory stage of any big data implementation with predictive analytics purposes. Extensive research on model selection and combination has revealed the benefits of such techniques in terms of forecast accuracy and reliability. Several criteria for model selection have been proposed and used for decades with very good results. Akaike information criterion and Schwarz Bayesian criterion are two of the most popular criteria. However, research on the combination of several criteria along with other sources of information in a unified methodology remains scarce.
This study proposes a new model selection approach that combines different criteria using a support vector machine (SVM). Given a set of candidate models, rather than considering any individual criterion, an SVM is trained at each forecasting origin to select the best model. This methodology will be particularly interesting for scenarios with highly volatile demand because it allows changing the model when it does not fit the data sufficiently well, thereby reducing the risk of misusing modeling techniques in the automatic processing of large datasets.
The effects of the proposed approach are empirically explored using a set of representative forecasting methods and a dataset of 229 weekly demand series from a leading household and personal care manufacturer in the UK. Our findings suggest that the proposed approach results in more robust predictions with lower mean forecasting error and biases than base forecasts.
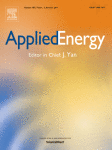
Is microbial fuel cell technology ready? An economic answer towards industrial commercialization
Juan R. Trapero and Laura Horcajada and Jose J. Linares and Justo Lobato
Applied Energy, 185: 698—707
Publication year: 2017
Over the last decade, Microbial Fuel Cells (MFCs) have experienced significant scientific and technological development, to the point of becoming close to commercialization. One key assessment that clearly establishes whether one technology can fully enter the market is the profitability demonstration. For this demonstration, classical evaluation criteria for investment decisions such as the Net Present Value and the Internal Rate of Return can be applied to a given proposal. This paper presents an economic assessment of a microbial fuel cell in a juice processing plant. Three different scenarios, optimistic, pessimistic and most likely scenarios based on the maximum power density of the cell on two basic MFC cases (cathodes with and without Pt, respectively), were studied and compared to the conventional activated sludge process. The results show that under most of the scenarios under consideration, including the pessimistic one, MFC is a more attractive option. Furthermore, a sensitivity analysis was performed with respect to the electrode area, and the annual growth rate of the electricity pricing has revealed that the electrode area parameter is the most influential, reducing the MFC profitability for larger electrode areas, whereas the higher the annual growth rates of the electricity price, the higher the MFC profits. In summary, the results of this study show that the implementation of MFC is a promising alternative to the use of classical aerated activated sludge, and it has potential economic benefits.
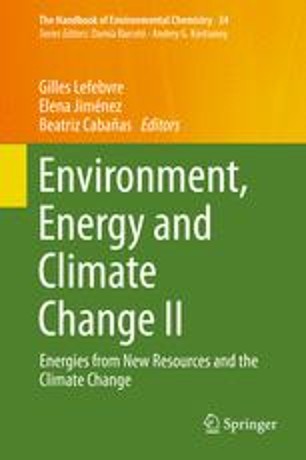
Recursive Estimation Methods to Forecast Short-Term Solar Irradiation
Martín, A. and Trapero, Juan R.
Environment, Energy and Climate Change II: Energies from New Resources and the Climate Change, 17-32
Publication year: 2016
Due to modern economies moving towards a more sustainable energy supply, solar power generation is becoming an area of paramount importance. In order to integrate this generated energy into the grid, solar irradiation must be forecasted, where deviations of the forecasted value involve significant costs. Intermittence, high frequency, and nonstationary are common features of solar irradiation data that have attracted the interest of numerous researchers from different disciplines. In fact, complex methods based on artificial intelligence have been typically used to address this problem. Nonetheless, adequate benchmarks have not been employed to justify such utilization. The objective of this work is to analyze the Holt–Winters (HW) method to forecast solar irradiation at short term (from 1 to 6 h). At the best of our knowledge, this methodology, which belongs to the family of the exponential smoothing methods and is widely used in industry applications ranging from supply chain demand forecasting to electricity load energy forecasting, has not been fully exploited in solar irradiation forecasting. Additionally, in case the accuracy achieved by the Holt–Winters method is not enough, still it can be utilized as a competitive benchmark given its feasible implementation. The Holt–Winters method performance is illustrated by forecasting two time series. Firstly, global horizontal irradiation (GHI) data have been chosen since forecasting GHI is a crucial part in systems based on photovoltaic (PV) energy conversion. Fluctuations of GHI due to passing clouds at short timescales (seconds and minutes) lead to high variability of power output from PV plants that can strain the grid due to voltage-flicker and balancing issues. Furthermore, direct normal irradiation (DNI) data are also forecasted given the role of main fuel that DNI plays in solar concentrator technologies such as solar thermal (CSP) and concentrated photovoltaic (CPV) power plants. Both solar irradiation data series have been hourly created from 1-minute irradiance measurements that were collected from ground-based weather stations located in Spain.
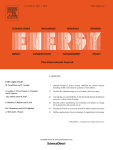
Calculation of solar irradiation prediction intervals combining volatility and kernel density estimates
Juan R. Trapero
Energy, 114: 266—274
Publication year: 2016
In order to integrate solar energy into the grid it is important to predict the solar radiation accurately, where forecast errors can lead to significant costs. Recently, the increasing statistical approaches that cope with this problem is yielding a prolific literature. In general terms, the main research discussion is centred on selecting the “best” forecasting technique in accuracy terms. However, the need of the users of such forecasts require, apart from point forecasts, information about the variability of such forecast to compute prediction intervals. In this work, we will analyze kernel density estimation approaches, volatility forecasting models and combination of both of them in order to improve the prediction intervals performance. The results show that an optimal combination in terms of prediction interval statistical tests can achieve the desired confidence level with a lower average interval width. Data from a facility located in Spain are used to illustrate our methodology.
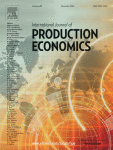
A novel time-varying bullwhip effect metric: An application to promotional sales
Juan R. Trapero and Diego J. Pedregal
International Journal of Production Economics, 182: 465—471
Publication year: 2016
Bullwhip effect is a problem of paramount importance that reduces competitiveness of supply chains around the world. A significant effort is being devoted by both practitioners and academics to understand its causes and to reduce its pernicious consequences. Nevertheless, limited research has been carried out to analyse potential metrics to measure it, that typically are summarized in the coefficient of variation ratio of different echelons demand. This work proposes a new metric based on a time-varying extension of the aforementioned bullwhip effect metric by employing recursive estimation algorithms expressed in the State Space framework to provide at each single time period a real-time bullwhip effect estimate. In order to illustrate the results, a case study based on a serially linked supply chain of two echelons from the chemical industry is analysed. Particularly, this metric is employed to analyse the effect of promotional campaigns on the bullwhip effect on a real-time fashion. The results show that, effectively, the bullwhip effect is not constant along time, but interestingly, it is reduced during the promotional periods and it is bigger before and after the promotion takes place.
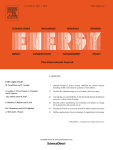
Short-term solar irradiation forecasting based on Dynamic Harmonic Regression
Juan R. Trapero and Nikolaos Kourentzes and A. Martin
Energy, 84: 289—295, 289-295
Publication year: 2015
Solar power generation is a crucial research area for countries that have high dependency on fossil energy sources and is gaining prominence with the current shift to renewable sources of energy. In order to integrate the electricity generated by solar energy into the grid, solar irradiation must be reasonably well forecasted, where deviations of the forecasted value from the actual measured value involve significant costs. The present paper proposes a univariate Dynamic Harmonic Regression model set up in a State Space framework for short-term (1–24 h) solar irradiation forecasting. Time series hourly aggregated as the Global Horizontal Irradiation and the Direct Normal Irradiation will be used to illustrate the proposed approach. This method provides a fast automatic identification and estimation procedure based on the frequency domain. Furthermore, the recursive algorithms applied offer adaptive predictions. The good forecasting performance is illustrated with solar irradiance measurements collected from ground-based weather stations located in Spain. The results show that the Dynamic Harmonic Regression achieves the lowest relative Root Mean Squared Error; about 30% and 47% for the Global and Direct irradiation components, respectively, for a forecast horizon of 24 h ahead.
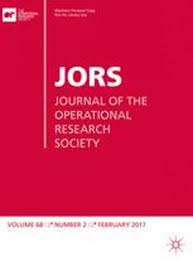
On the identification of sales forecasting models in the presence of promotions
Trapero, J.R. and Kourentzes, N. and Fildes, R.
Journal of the Operational Research Society, 66(2), 299–307
Publication year: 2015
Shorter product life cycles and aggressive marketing, among other factors, have increased the complexity of sales forecasting. Forecasts are often produced using a Forecasting Support System that integrates univariate statistical forecasting with managerial judgment. Forecasting sales under promotional activity is one of the main reasons to use expert judgment. Alternatively, one can replace expert adjustments by regression models whose exogenous inputs are promotion features (price, display, etc). However, these regression models may have large dimensionality as well as multicollinearity issues. We propose a novel promotional model that overcomes these limitations. It combines Principal Component Analysis to reduce the dimensionality of the problem and automatically identifies the demand dynamics. For items with limited history, the proposed model is capable of providing promotional forecasts by selectively pooling information across established products. The performance of the model is compared against forecasts provided by experts and statistical benchmarks, on weekly data; outperforming both substantially.
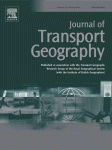
Measuring the substitution effects between High Speed Rail and air transport in Spain
José I. Castillo-Manzano and Rafael Pozo-Barajas and Juan R. Trapero
Journal of Transport Geography, 43: 59—65
Publication year: 2015
The main objective of this paper is to estimate the impact that the expansion of the HSR network has had on air transport in Spain by estimating the substitution effect between the two types of transportation. This paper considers the way that the HSR network has grown and how this growth could have affected air transport dynamically. The findings show that a dynamic vision of this substitution rate should be adopted, as opposed to assuming that the rate is constant, as has been the case in previous references. Although the rate varies significantly over the study period, only 13.9% of HSR passenger demand was found to have come from air travel during the 1999–2012 period, meaning that HSR and airlines would seem to offer more independent services than at first it might appear. This confirms the hypothesis as to the HSR’s great ability to generate its own demand. The substitution rate between the two transport modes seems to be closely linked to the way that any new stations are incorporated into the HSR network. Convergence between the seasonality of HSR and air transport has also been examined. The results show that it is difficult to talk of a real HSR transport network in Spain.
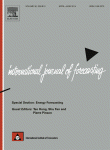
Book review Recursive Estimation and Time-Series Analysis. An Introduction for the Student and Practitioner, Second edition, Springer (2011),
Juan R. Trapero
Volume 31, Issue 1, January–March 2015, 91-92
Publication year: 2015
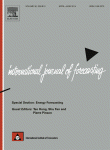
Improving forecasting by estimating time series structural components across multiple frequencies
Kourentzes, N. and Petropoulos, F. and Trapero, J.R.
International Journal of Forecasting, 30(2), 291-302
Publication year: 2014
Identifying the most appropriate time series model to achieve a good forecasting accuracy is a challenging task. We propose a novel algorithm that aims to mitigate the importance of model selection, while increasing the accuracy. Multiple time series are constructed from the original time series, using temporal aggregation. These derivative series highlight different aspects of the original data, as temporal aggregation helps in strengthening or attenuating the signals of different time series components. In each series, the appropriate exponential smoothing method is fitted and its respective time series components are forecast. Subsequently, the time series components from each aggregation level are combined, then used to construct the final forecast. This approach achieves a better estimation of the different time series components, through temporal aggregation, and reduces the importance of model selection through forecast combination. An empirical evaluation of the proposed framework demonstrates significant improvements in forecasting accuracy, especially for long-term forecasts.
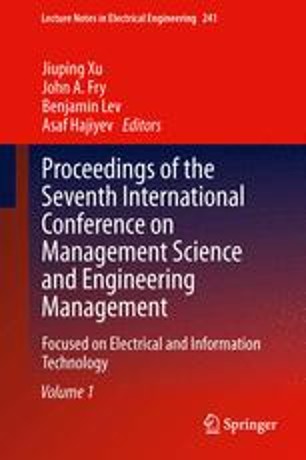
Impact of Demand Nature on the Bullwhip Effect. Bridging the Gap between Theoretical and Empirical Research
Trapero, Juan R. and García, Fausto P. and Kourentzes N.
In Proceedings of the Seventh International Conference on Management Science and Engineering Management, 1127-1137
Publication year: 2014
The bullwhip effect (BE) consists of the demand variability amplification that exists in a supply chain when moving upwards. This undesirable effect produces excess inventory and poor customer service. Recently, several research papers from either a theoretical or empirical point of view have indicated the nature of the de- mand process as a key aspect to defining the BE. Nonetheless, they reached different conclusions. On the one hand, theoretical research quantified the BE depending on the lead time and ARIMA parameters, where ARIMA functions were employed to model the demand generator process. In turn, empirical research related nonlinearly the demand variability extent with the BE size. Although, it seems that both results are contradictory, this paper explores how those conclusions complement each other. Essentially, it is shown that the theoretical developments are precise to determine the presence of the BE based on its ARIMA parameter estimates. Nonetheless, to quan- tify the size of the BE, the demand coefficient of variation should be incorporated. The analysis explores a two-staged serially linked supply chain, where weekly data at SKU level from a manufacturer specialized in household products and a major UK grocery retailer have been collected.
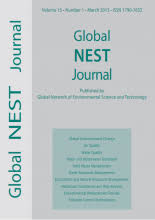
A novel approach to fault detection and diagnosis on wind turbines
Gómez, C.Q. and Ruíz de la Hermosa, R. and Trapero, J. R. and García, F. P.
Global NEST JournalGlobal NEST: the international Journal, 16(6), 1029 – 1037
Publication year: 2014
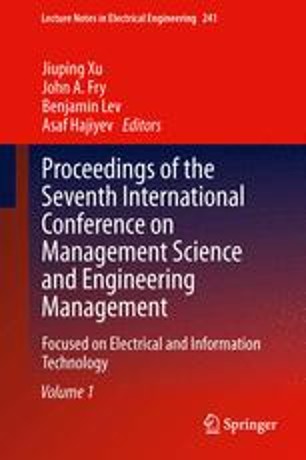
A New Ranking Method Approach for Decision Making in Maintenance Management
García Márquez, Fausto, and Pliego, Alberto and Lorente, José and Trapero, Juan R.
In Proceedings of the Seventh International Conference on Management Science and Engineering Management, 27-38
Publication year: 2014
Decision making process in maintenance management produces a final choice. Fault Tree Analysis (FTA) is proposed as a graphical representation of logical relationships between the elements that comprise the decision making process in maintenance management. A Fault Tree (FT) is compound by different events and logic gates. Complex systems analysis may produce thousands of combinations of events (cut-sets) that can cause the system failure. The determination of these cut-sets can be a large and time-consuming process even on modern computers. Binary Decision Diagrams (BDD) provides a new alternative to the traditional cut-set based approach for FTA that leads to the determination of the function output value through the examination of the input values. BDD is a directed acyclic graph that represents the Boolean functions. The cut sets generated by BDD will depend on the events ordering. The “Level”, “Top-Down-Left–Right”, “AND”, “Depth-First Search” and “Breadth-First Search” methods have been considered for listing the events, and a comparative analysis of them has been done. A new ranking approach is proposed in this paper, where its efficiency has been validated.
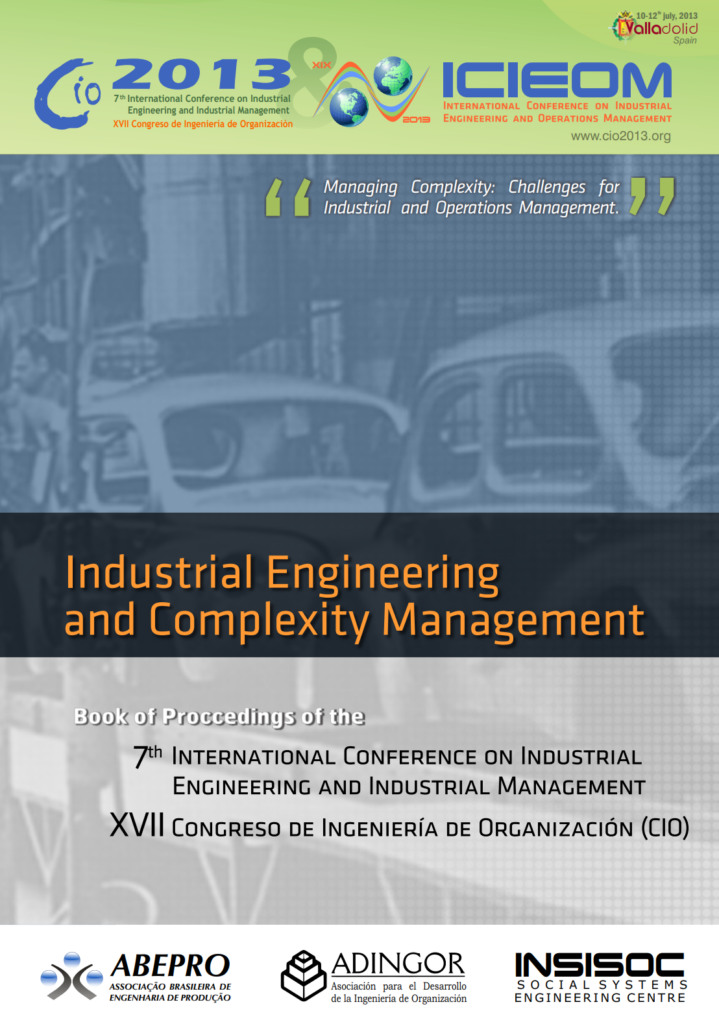
Técnicas de predicción cuantitativas aplicadas a la cadena de suministro. Un caso de estudio
Trapero, Juan R. and García, Fausto P.
The 7th International Conference on Industrial Engineering and Industrial Management., 1016-1024
Publication year: 2013
Demand forecasting is a complex topic due to different factors like promotions. Generally, promotions may be forecast by using an univariate statistical approach (system forecast) that is judgmentally adjusted by company experts. The present work reports an analysis of the managerial adjustments accuracy when promotions are taking place. Additionally, quantitative models will be assessed as an alternative to judgmental adjustments when referring to forecast promotions. The results show that judgmentally adjusted forecasts on promotion periods may enhance system forecasts, but not systematically and more importantly, multivariate models based on past promotions information might achieve lower forecasting errors than system and judgmentally adjusted forecasts.
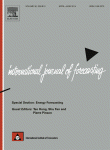
Analysis of judgmental adjustments in the presence of promotions
Trapero, J.R. and Pedregal, D.J. and Fildes, R. and Kourentzes, N.
International Journal of Forecasting, 29(2), 234-243
Publication year: 2013
Sales forecasting is becoming increasingly complex, due to a range of factors, such as the shortening of product life cycles, increasingly competitive markets, and aggressive marketing. Often, forecasts are produced using a Forecasting Support System that integrates univariate statistical forecasts with judgment from experts in the organization. Managers then add information to the forecast, such as future promotions, potentially improving the accuracy. Despite the importance of judgment and promotions, papers devoted to studying their relationship with forecasting performance are scarce. We analyze the accuracy of managerial adjustments in periods of promotions, based on weekly data from a manufacturing company. Intervention analysis is used to establish whether judgmental adjustments can be replaced by multivariate statistical models when responding to promotional information. We show that judgmental adjustments can enhance baseline forecasts during promotions, but not systematically. Transfer function models based on past promotions information achieved lower overall forecasting errors. Finally, a hybrid model illustrates the fact that human experts still added value to the transfer function models.
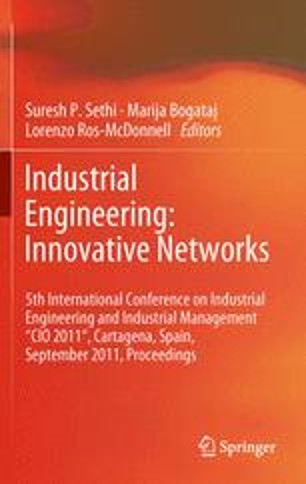
The Power of ECOTOOL MATLAB Toolbox
Pedregal, Diego J and Trapero, Juan R.
In: Sethi S., Bogataj M., Ros-McDonnell L. (eds) Industrial Engineering: Innovative Networks, 319-327
Publication year: 2012
This paper presents the ECOnometrics TOOLbox (ECOTOOL), a new MATLAB forecasting toolbox that embodies several tools for identification, validation and forecasting of dynamic models based on time series analysis. Tools to perform a wide range of exploratory and statistical tests with visual counterparts are included, designed in easy-to-use front ends. The models implemented so far are classical in essence (in the very first version of the toolbox), among them, ARIMA, Exponential Smoothing, Unobserved Components, ARX, ARMAX, Transfer Function, Dynamic Regression and Distributed Lag models. The main idea of this development is providing a tool that, with a few code lines, performs a great number of tasks. The toolbox is presented in all its potentiality on the Spanish Industrial Production Index.
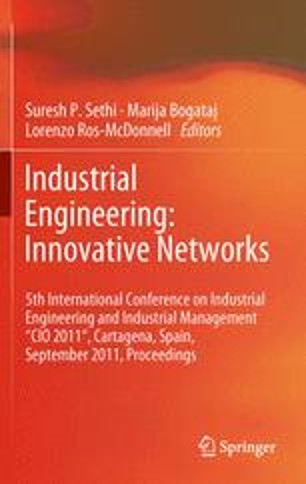
Supply Chain Demand Forecasting: Towards an Integrated Approach
Trapero, Juan R. and Pedregal, Diego J.
In Ïndustrial Engineering: Innovative Networks, 275-282
Publication year: 2012
Demand forecasts are crucial to drive supply chains and enterprise resource planning systems. Improved accuracy in forecasts directly affects all levels of the supply chain, reducing stock costs and increasing customer satisfaction. Usually, this problem is faced by testing various time series methods with a different level of complexity to find out which one is the most accurate. From our point of view, the problem should be re-addressed. In this sense, the effort should be focused on incorporating more efficient sources of information that are frequently overlooked. This paper explores different sources of information (apart from past observations) that might enhance the capability of a company to produce accurate forecasts. Such sources are: (i) Judgmental forecasting at SKU level and (ii) Information sharing. Additionally, new models are proposed to integrate such information well. Data collected from a manufacturer of household cleaning products and a major UK grocery retailer are used to illustrate the procedure.
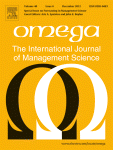
Impact of information exchange on supplier forecasting performance
Juan R. Trapero and N. Kourentzes and R. Fildes
Omega, 40(6): 738—747
Publication year: 2012
Forecasts of demand are crucial to drive supply chains and enterprise resource planning systems. Usually, well-known univariate methods that work automatically such as exponential smoothing are employed to accomplish such forecasts. The traditional Supply Chain relies on a decentralized system where each member feeds its own Forecasting Support System (FSS) with incoming orders from direct customers. Nevertheless, other collaboration schemes are also possible, for instance, the Information Exchange framework allows demand information to be shared between the supplier and the retailer. Current theoretical models have shown the limited circumstances where retailer information is valuable to the supplier. However, there has been very little empirical work carried out. Considering a serially linked two-level supply chain, this work assesses the role of sharing market sales information obtained by the retailer on the supplier forecasting accuracy. Weekly data from a manufacturer and a major UK grocery retailer have been analyzed to show the circumstances where information sharing leads to improved forecasting accuracy. Without resorting to unrealistic assumptions, we find significant evidence of benefits through information sharing with substantial improvements in forecast accuracy.
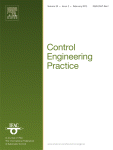
Adaptive input shaping for single-link flexible manipulators using an algebraic identification
Pereira, E. and Trapero, J.R. and Díaz, I.M. and Feliu, V.
Control Engineering Practice, 20(2) 138-147
Publication year: 2012
This work proposes an adaptive control scheme applied to single link-flexible manipulators, which combines a feedback controller of the joint angle with an adaptive input shaper updated by an algebraic non-asymptotic identification. The feedback controller is designed to guarantee trajectory tracking of the joint angle, simplifying thus the input shaper, which can be designed for the arm dynamics only. The input shaper is updated by an algebraic identification of the natural frequency corresponding to the first vibration mode of the arm. In addition, the influence of the assumptions adopted to derive the algebraic identification on the performance of the estimation is studied. Finally, the proposed adaptive control strategy is implemented in practice.
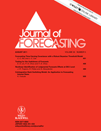
Nonlinear identification of judgmental forecasts effects at SKU level
Trapero, J.R. and Fildes, R. and Davydenko, A.
Journal of Forecasting, 30(5), 490-508
Publication year: 2011
Prediction of demand is a key component within supply chain management. Improved accuracy in forecasts directly affects all levels of the supply chain, reducing stock costs and increasing customer satisfaction. In many application areas, demand prediction relies on statistical software which provides an initial forecast subsequently modified by the expert’s judgment. This paper outlines a new methodology based on state‐dependent parameter (SDP) estimation techniques to identify the nonlinear behaviour of such managerial adjustments. This non‐parametric SDP estimate is used as a guideline to propose a nonlinear model that corrects the bias introduced by the managerial adjustments. One‐step‐ahead forecasts of stock‐keeping unit sales sampled monthly from a manufacturing company are utilized to test the proposed methodology. The results indicate that adjustments introduce a nonlinear pattern, undermining accuracy. This understanding can be used to enhance the design of the forecasting support system in order to help forecasters towards more efficient judgmental adjustments. Copyright © 2010 John Wiley & Sons, Ltd.
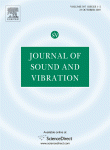
An adaptive pneumatic suspension based on the estimation of the excitation frequency
Nieto, A.J. and Morales, A.L. and Trapero, J.R. and Chicharro, J.M. and Pintado, P.
Journal of Sound and Vibration, 330(9), 1891-1903
Publication year: 2011
A pneumatic suspension that can adapt itself to the incoming vibration is presented in this paper. A switching control strategy between two different configurations is proposed and studied. The objective is to avoid undesirable resonant frequencies. The control procedure is based on the pre-knowledge of the incoming vibration frequency, and when this frequency is unknown, a very efficient prediction technique is used. The results show that the adaptable suspension has improved performance as compared to any of its passive counterparts. The transient response when switching typically takes less than three cycles and does not hinder the suspension performance.
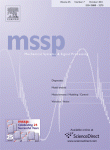
Algebraic identification of the first two natural frequencies of flexible-beam-like structures
Pereira, E. and Trapero, J.R. and D’iaz, I.M. and Feliu, V.
Mechanical Systems and Signal Processing, 25(7), 2324-2335
Publication year: 2011
The residual vibrations of highly resonant flexible structures can be usually composed of sinusoidal signals. The identification of the frequencies, amplitudes and/or phases of these sinusoidal signals may be of interest for health monitoring or to update controller parameters in active vibration control systems. This work considers a previously developed algebraic estimator of the frequencies, amplitudes and phases of two sinusoidal signals in order to identify the first two natural frequencies of flexible-beam-like structures. The influence of the unconsidered vibration modes on the estimation accuracy is studied by simulating a residual vibration formed by four sinusoidal components. It has been shown that the velocity and accuracy in the estimation of the first two natural frequencies depend on the distance between frequencies and the amplitudes of the first three vibration components. Finally, the beam parameters are related to the estimation performance.
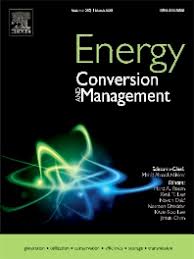
Mid-term hourly electricity forecasting based on a multi-rate approach
Pedregal, D.J. and Trapero, J.R.
Energy Conversion and Management, 51(1), 105-111
Publication year: 2010
Load demand prediction for mid or long-term horizons is important for the development of any model for electric power system planning. Literature on this topic is much scarcer than short-term forecasting, mainly due to the inherent difficulties in long-term modelling. The aim of this paper is to develop a general multi-rate methodology in order to forecast optimally load demand series sampled at an hourly rate for a mid-term horizon. This method may be considered as an extension of a previously published short-term approach to predict load and prices based on unobserved components. This approach implies the estimation of different models for the same data sampled at different rates (monthly and hourly in this paper). Each model incorporates the appropriate features of the data for its respective sampling interval, and both types of forecasts are integrated in one single forecast by efficient time aggregation techniques that result natural to implement in a State Space framework. The procedure is evaluated by a thorough forecasting experiment in which 365 rolling sets of one hour up to 12 weeks ahead of hourly forecasts are produced for the load demand registered at a transformer of a UK company. The results show that this method produces a notable reduction on the prediction error and its variability.
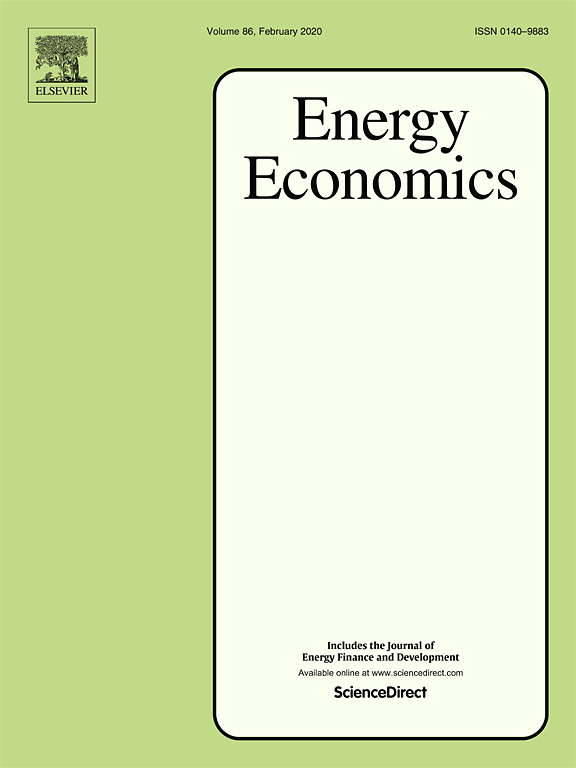
Frequency domain methods applied to forecasting electricity markets
Trapero, J.R. and Pedregal, D.J.
Energy Economics, 31(5), 727-735
Publication year: 2009
The changes taking place in electricity markets during the last two decades have produced an increased interest in the problem of forecasting, either load demand or prices. Many forecasting methodologies are available in the literature nowadays with mixed conclusions about which method is most convenient. This paper focuses on the modeling of electricity market time series sampled hourly in order to produce short-term (1 to 24 h ahead) forecasts. The main features of the system are that (i) models are of an Unobserved Component class that allow for signal extraction of trend, diurnal, weekly and irregular components; (ii) its application is automatic, in the sense that there is no need for human intervention via any sort of identification stage; (iii) the models are estimated in the frequency domain; and (iv) the robustness of the method makes possible its direct use on both load demand and price time series. The approach is thoroughly tested on the PJM interconnection market and the results improve on classical ARIMA models.
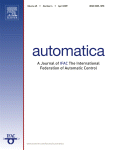
Adaptive input shaping for manoeuvring flexible structures using an algebraic identification technique
Pereira, E. and Trapero, J.R. and Díaz, I.M. and Feliu, V.
Automatica, 45(4), 1046-1051
Publication year: 2009
Input shaping is an efficient feedforward control technique which has motivated a great number of contributions in recent years. Such a technique generates command signals with which manoeuvre flexible structures without exciting their vibration modes. This paper presents a novel adaptive input shaper based on an algebraic non-asymptotic identification. The main characteristic of the algebraic identification in comparison with other identification methods is the short time needed to obtain the system parameters without defining initial conditions. Thus, the proposed adaptive control can update the input shaper during each manoeuvre when large uncertainties are present. Simulations illustrate the performance of the proposed method.
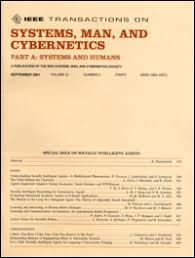
Adaptive controller for single-link flexible manipulators based on algebraic identification and generalized proportional integral control
Becedas, J. and Trapero, J.R. and Feliu, V. and Sira-Ramírez, H.
IEEE Transactions on Systems, Man, and Cybernetics, Part B: Cybernetics, 39(3), 735 – 751
Publication year: 2009
In this paper, we propose a fast online closed-loop identification method combined with an output-feedback controller of the generalized proportional integral (GPI) type for the control of an uncertain flexible robotic arm with unknown mass at the tip, including a Coulomb friction term in the motor dynamics. A fast nonasymptotic algebraic identification method developed in continuous time is used to identify the unknown system parameter and update the designed certainty equivalence GPI controller. In order to verify this method, several informative simulations and experiments are shown.
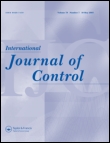
On the algebraic identification of the frequencies, amplitudes and phases of two sinusoidal signals from their noisy sum
Trapero, J.R. and Sira-Ramírez, H. and Batlle, V.F.
International Journal of Control, 81(3), 507-518
Publication year: 2008
An algebraic identification approach is used for the fast and reliable on-line determination of the defining parameters of two sinusoidal signals of different, unknown, amplitudes, phases and frequencies from their noise-perturbed measured sum. The proposed method is based on the algebraic derivative approach, defined in the frequency domain, yielding exact calculation formulae for the unknown parameters when interpreted in a noise-free time-domain environment. The proposed computation formulae are synthesized in terms of time-varying linear, unstable, filters in combination with classical low-pass filters. The proposed algorithms are insensitive to initial conditions, require no special design parameter tuning and the fast convergence is of non-asymptotic nature. The on-line computations are performed in a time interval which is only a fraction of the first full cycle of one of the integrating components of the measured signal. Several simulations are shown to verify the algorithm proposed. Finally, experimental results dealing with actual laboratory signals are presented.
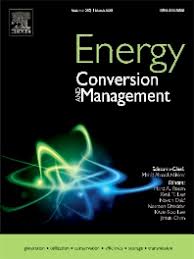
Electricity prices forecasting by automatic dynamic harmonic regression models
Pedregal, D.J. and Trapero, J.R.
Energy Conversion and Management, 48(5), 1710-1719
Publication year: 2007
The changes experienced by electricity markets in recent years have created the necessity for more accurate forecast tools of electricity prices, both for producers and consumers. Many methodologies have been applied to this aim, but in the view of the authors, state space models are not yet fully exploited. The present paper proposes a univariate dynamic harmonic regression model set up in a state space framework for forecasting prices in these markets. The advantages of the approach are threefold. Firstly, a fast automatic identification and estimation procedure is proposed based on the frequency domain. Secondly, the recursive algorithms applied offer adaptive predictions that compare favourably with respect to other techniques. Finally, since the method is based on unobserved components models, explicit information about trend, seasonal and irregular behaviours of the series can be extracted. This information is of great value to the electricity companies’ managers in order to improve their strategies, i.e. it provides management innovations. The good forecast performance and the rapid adaptability of the model to changes in the data are illustrated with actual prices taken from the PJM interconnection in the US and for the Spanish market for the year 2002.
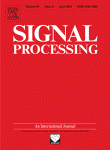
An algebraic frequency estimator for a biased and noisy sinusoidal signal
Trapero, J.R. and Sira-Ramírez, H. and Batlle, V.F.
Signal Processing, 87(6), 1188-1201
Publication year: 2007
An algebraic approach is proposed for the fast and reliable, on line, identification of the amplitude, frequency and phase parameters in unknown noisy sinusoidal signals. The proposed method uses the algebraic derivative method in the frequency domain yielding exact formulae, when placed in the time domain, for the unknown parameters. These formulae are synthesized in terms of time-varying linear unstable filters in combination with classical low-pass filters of the Butterworth type. The computations are performed in a quite small time interval which is only a small fraction of the first full cycle of the measured sinusoid signal. The method is also verified to be robust with respect to signal measurement noises and structured perturbations via digital computer simulations and, also, via actual laboratory experiments.
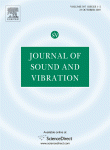
A fast on-line frequency estimator of lightly damped vibrations in flexible structures
Trapero, J.R. and Sira-Ramírez, H. and Batlle, V.F.
Journal of Sound and Vibration, 307(1-2), 365-378
Publication year: 2007
A significant research effort has been conducted in the past to achieve a reliable on-line vibration mode estimator. Frequently, pure sinusoidal models of the underlying mechanical system have been used to accomplish the estimation task. In this paper, an algebraic approach is proposed for the fast and reliable, on-line identification of the natural frequency of a flexible-link manipulator. The proposed method uses the pure sinusoidal model in combination with the algebraic derivative method for parameter identification. The method leads, in the time domain, to an exact computation formulae for the unknown frequency parameter. This formula is synthesized in terms of time-varying linear unstable filters in combination with classical low-pass filters of the Butterworth type. The computations are performed in a quite small-time interval which is only a small fraction of the first full cycle of the measured sinusoidal signal. The proposed method is verified to be robust with respect to un-modeled small attenuations, present in the flexible structure, and to measurement noise. An experimental setup has been developed as a benchmark to test and compare the proposed method with other recently developed parameter estimation techniques.